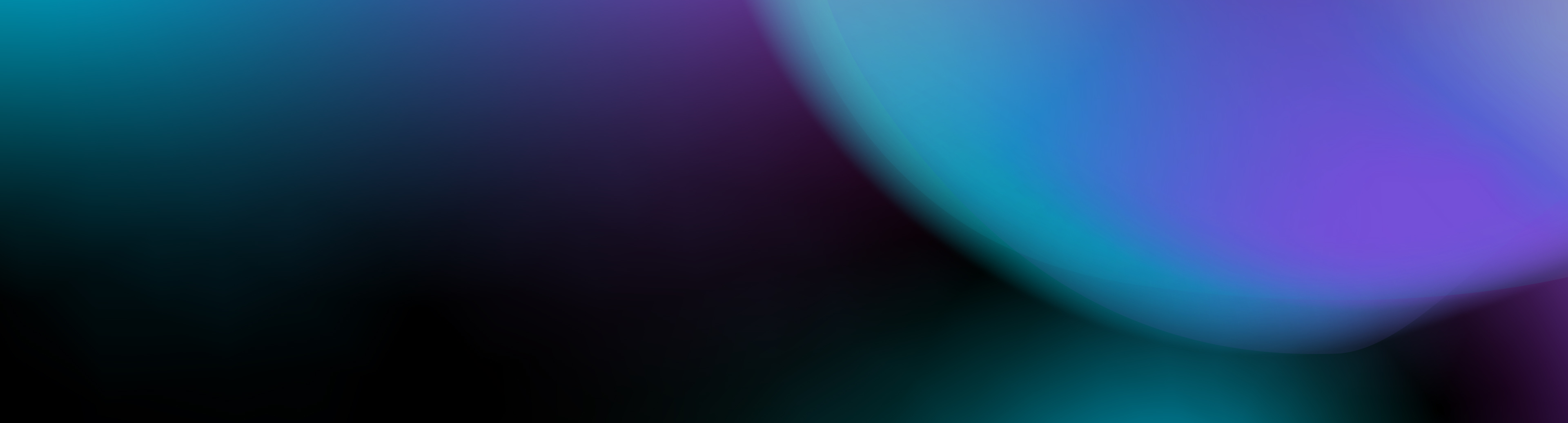
Fighting climate change with climate data
How French startup the climate data factory is helping organizations take actionable steps to forecast and battle the effects of climate change.
How French startup the climate data factory is helping organizations take actionable steps to forecast and battle the effects of climate change.
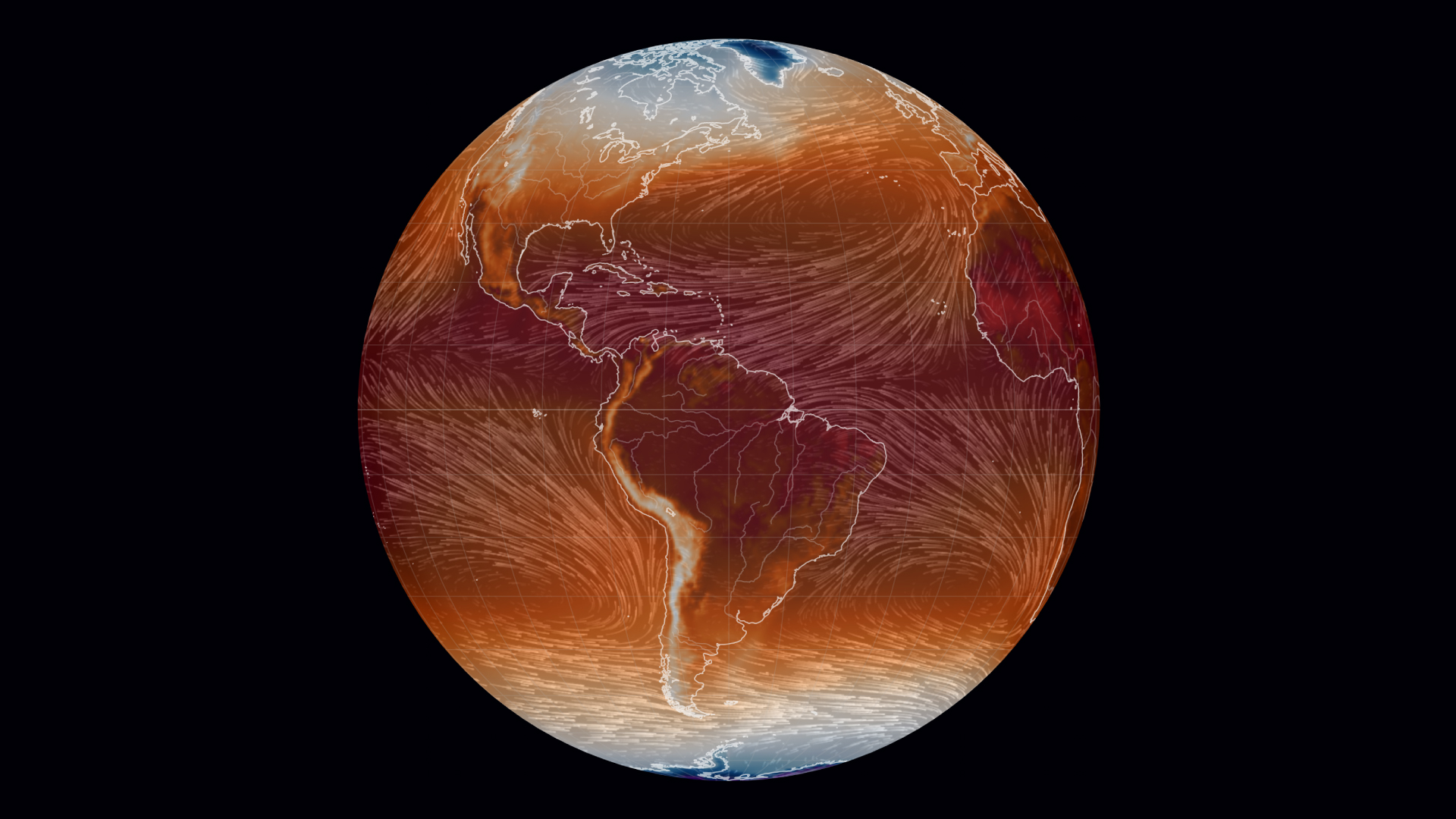
A new AI framework processes climate datasets to improve the detection of extreme weather events. Photo source: the climate data factory
The summary document for climate policy makers released by the Intergovernmental Panel on Climate Change (IPCC) starts with a sobering announcement: “It is unequivocal that human influence has warmed the atmosphere, ocean and land. Widespread and rapid changes in the atmosphere, ocean, cryosphere and biosphere have occurred… Each of the last four decades has been successively warmer than any decade that preceded it since 1850.”
Policy makers such as the ones at the IPCC are increasingly turning to machine learning to fight the impacts of climate change. Organizations around the world are increasingly turning to machine learning to improve or find new ways to better understand climate and its impacts. the climate data factory is a French startup making future climate information easily accessible. Harilaos Loukos, the CEO of the climate datafactory believes that enabling access to actionable climate data is essential if we are to make informed and effective climate actions.
Loukos is an entrepreneur with an extensive background in climate research. Prior to the climate data factory, Loukos was the CEO and co-founder of Climpact-Metnext, a startup that provided operational tools and services to measure weather impacts on economic activity. The company was acquired by Weathernews in 2017.
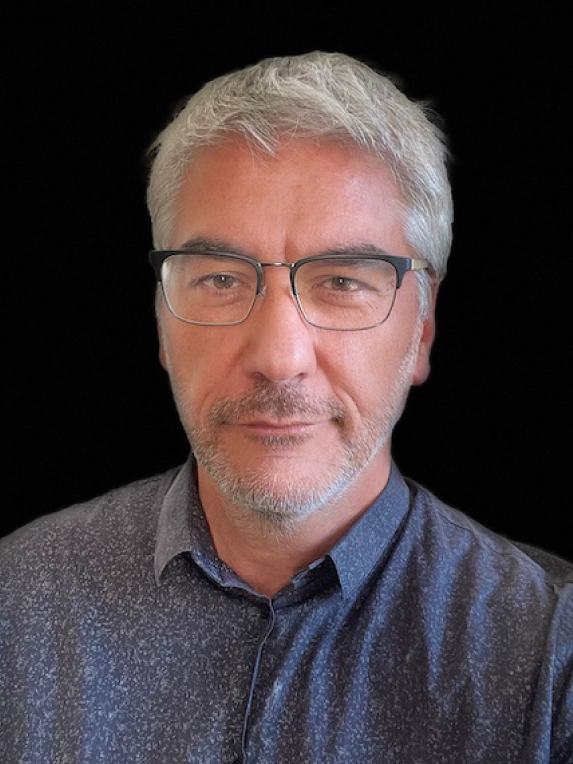
In a conversation with Amazon re:MARS, Loukos spoke about climate modeling, the importance of climate projections at local spatial resolutions and CLINT (short for climate intelligence), a new AI framework of machine learning techniques and algorithms to process big climate datasets for improving the detection, causation and attribution of extreme weather events.
What is the kind of data available on the climate data factory?
We have two kinds of model data. The first kind is related to climate projections; they are produced by research organizations around the world and used to forecast changes in weather patterns due to man-made greenhouse gas emissions. This data is at the base of scientific results that support international climate negotiations—nations arriving at agreements on future emissions at international climate accords like the 2015 Paris Agreement. More recently, this data is also being used to support local risk assessment studies to plan for adaptation strategies in a changing climate. And here’s where we can add a lot of value at the climate data factory. Climate projections from global climate models typically provide resolutions of around 10,000 square kilometers. However, when adaptation strategies are developed at local scales—for example, by a city government—they require projections at much smaller spatial resolutions.
The second kind data helps enable long term forecasts. We get these “climate forecasts” from authoritative operational sources like the European Centre for Medium-Range Weather Forecasts (ECMWF) and the Copernicus Climate Change Service (C3S). Think of this data enabling something akin to a weather forecast, but four weeks out (a subseasonal forecast) or even three to six months out (a seasonal forecast). ‘Til recently, these types of forecasts have largely played out in the experimental level of thought. However, due to the development of more sophisticated models (including the ocean and other climate components) that are operated in a multi-model set-up (i.e., different prediction systems) and a probabilistic approach (many forecast from each model) they can be much more accurate today.
If you are not producing the data, what is the added value that you provide to organizations?
We focus on the management and processing of these datasets. We provide the skills, resources and know-how to make this data ready, reliable and applicable for downstream applications. For example, we use statistical techniques to increase the spatial resolution of climate projections from 100 to 200 km to a more focused 10 to 25 km so that they can be used for local-level studies. For the longer-term forecasts, we apply statistical calibration techniques using observations to enhance the accuracy of the forecasts.
Can you give me a concrete example of how an organization can use these models to arrive at a concrete decision?
Our high-resolution climate projections are used by consultants to help local authorities develop effective climate adaptation strategies. In the private sector, financial and insurance institutions in North America and Europe use climate projections data to comply with mandatory physical risk assessments regarding climate change. Our models related to climate forecasts are used to make sector specific decisions such as renewable energy forecasting. We provide our climate forecast to decision support tools that help green energy professionals make decisions on how weather patterns translate into energy forecasts. In short, we make it easy for public and private sector organizations develop and extend their projects, services or applications with climate model data.
What is novel about the algorithms you use to power the climate data factory?
On the engineering front, our teams are working on code optimization, resource allocation, and developing quality control procedures to provide the best possible data service. On the scientific front, we stay current with the evolution of processing techniques by following technical advances in the scientific literature. We also collaborate extensively with academic institutions to guarantee high standards of processing. Transparency is critical when it comes to climate modeling, and we make sure our methods and datasets are published in reputed venues and journals. To give just one example, we recently published a paper outlining how we use sophisticated quantile mapping to arrive at a high-resolution climate projections dataset.
What is the Climate Intelligence Project (CLINT)?
With the massive amount of climate-related data, the use of artificial intelligence and machine learning is growing rapidly, and many large organizations like the National Oceanic and Atmospheric Administration (NOAA), and the ECMWF now have an AI/ML strategy.
We have recently launched a EU funded four-year international research project named CLINT in collaboration with 15 academic institutions (from Italy, Spain, Germany, Belgium and the Netherlands), private institutions (in France and Greece), national weather services (from Sweden and Germany) and ECMWF. The objective of CLINT is the development of an AI framework of machine learning techniques and algorithms to process big climate datasets for improving the detection (spatial patterns), causation (the physical triggers), and attribution (links with climate change) of extreme events including tropical cyclones, heatwaves, and extreme droughts.
Extreme events like the heat dome in North America or the drought and wildfires in California have immense repercussions on the day-to-day life of people. In Europe, we like to think of this impact occurring at the water-energy-food nexus—where a severe weather event can have impacts on all these three pillars of society. Take the example of this summer of combined drought/wildfires and floods in Europe—with CLINT, we aim at better forecasting not only the occurrence of these extreme events, but also support strategies to minimize their impact on members of society. We’ll take this approach to climate modeling one step further with CLINT—we will also develop a public facing “demonstrator” and a software library to facilitate the uptake of project results by researchers in public and private entities.