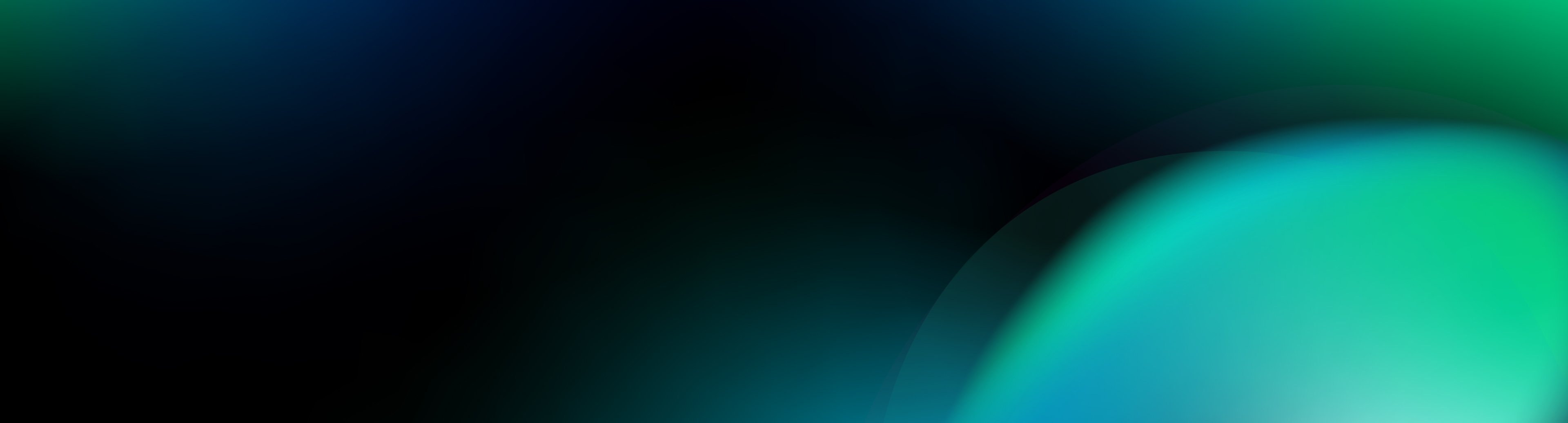
Artificial intelligence has a future in studying the past
Automation and AI are empowering archaeologists to make remarkable discoveries.
Automation and AI are empowering archaeologists to make remarkable discoveries.
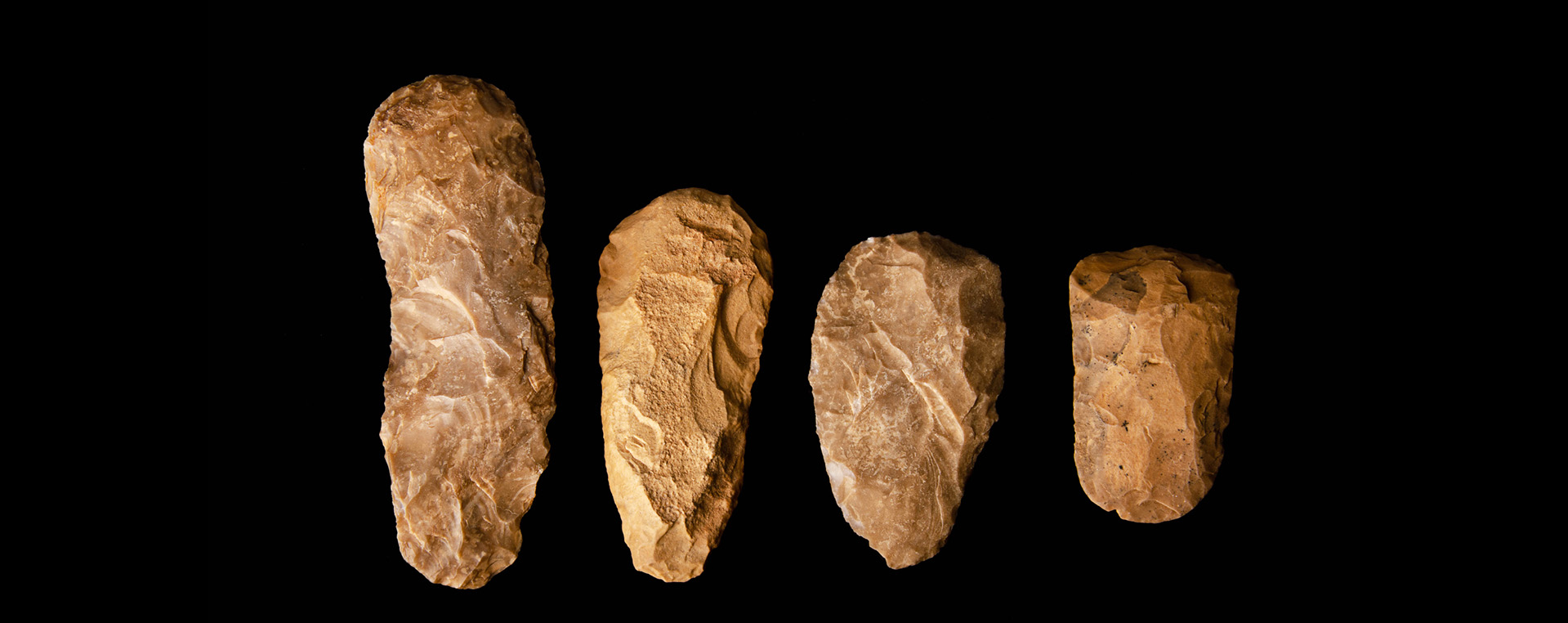
Modern technology is helping scientists better understand our past. Photo source: Shutterstock
Thousands of years ago, ancient globalization changed the face of cultures from Greece to Mesopotamia. As contact grew between diverse cultures, the faces of statues in far-flung cities grew more similar. Artificial intelligence recently helped archaeologists compare image data to prove that sculptures from distant areas show an increasing similarity.
University College London archaeologist Mark Altaweel and Ludwig Maximilian University of Munich historian Andrea Squitieri used a “hashing” algorithm to compare the facial features of statues made in countries around the Mediterranean, northern Africa, and Mesopotamia starting in the Bronze Age. The technology which is similar to common facial recognition programs, showed that from about the 800s BCE onward, statue faces got more and more similar over a wide geographic area.
That suggests people were sharing ideas and worldviews across thousands of miles. “This is true in a variety of things such as religion, language, various cultural processes, etc.,” Altaweel said in an email. “Empires were the key to this, along with population movement.”
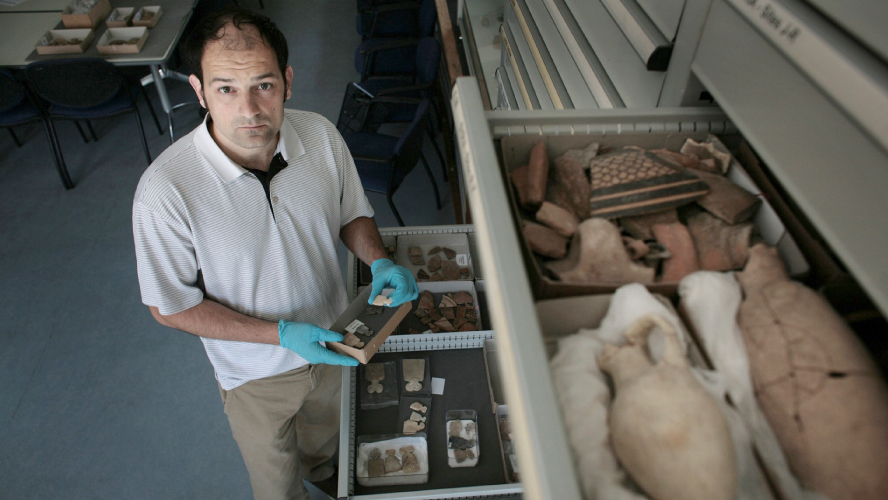
Earlier in their research, Altaweel and Squitieri had already noticed the pattern, but machine learning helped them describe and measure it.
“Now we have numbers to back it up,” Altaweel said. “We have a way to quantify that similarity, and that is key to making something that was previously not very scientific (comparing objects manually or visually) to something that is more scientific (having a machine come up with a value of similarity).”
Finding long-lost sites
But before archaeologists can understand how trade and conquest linked ancient societies, they have to find evidence of these cross-linkages in sites and artifacts. From the deserts of Peru to the steppes of China, archaeologists are using algorithms to search aerial and satellite images for the faint outlines of burial mounds, ancient villages, and other traces of the past. Data from satellites and the growing prevalence of drones have fueled the use of artificial intelligence in archaeology.
In 2019, Yamagata University archaeologist Masato Sakai and his colleagues trained a machine learning algorithm to recognize geoglyphs in Peru’s arid Nazca desert: huge drawings etched into the floor of the desert around 2,000 years ago. The famous Nazca Lines consist of more than 900 drawings of animals, people, and geometric designs.
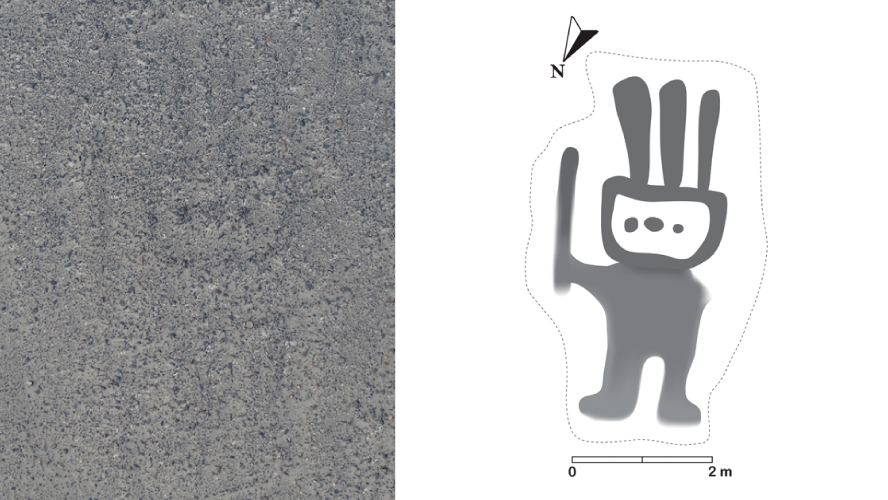
After learning to recognize the shapes, patterns of contrast between light and dark, and other features that make a geoglyph stand out from the empty desert floor, the algorithm found the faint outline of a five-meter-long humanoid figure wearing a headdress and carrying a stick. Sakai and his colleagues hiked into the desert to see the ancient artwork in person and confirmed that the AI was correct.
Seeing the big picture
Aerial and satellite photos can also help archaeologists study the big picture of the past: how long-ago settlements were connected, how people settled or migrated across landscapes, and how they interacted across large distances.
As Dylan S. Davis and other researchers explain, most archaeological research happens at individual sites. But that’s a bit like trying to understand 21st-century midwestern US culture by excavating one neighborhood in Nebraska. To understand the past on a larger scale, archaeologists need a wider view.
In 2018, Davis and colleagues programmed a machine learning algorithm to look for shell rings using LiDAR (an imaging method that works like radar, but uses reflected laser beams instead of radio waves) imagery of the South Carolina coast. They were looking for rings of oyster shells, discarded en masse by groups of ancient Native Americans. At some sites, people had piled discarded shells high enough to form tall rings around the outskirts of ancient towns or villages. However, at other sites, the shell rings seem to mark the places people only visited for feasts and other gatherings.
The team’s algorithm used object-based image analysis, or OBIA, to detect shell rings. The algorithm analyzed images to correlate patterns of pixels to corresponding objects. Human observers can spot the general shape of a shell ring. However, an OBIA algorithm can go further by searching for specific patterns of contrasting pixels, allowing it to spot sites a person would miss. The AI-assisted search helped researchers identify a 3,000 year-old shell ring that had previously been undetected.
Elsewhere, a 2019 survey used a deep learning algorithm called a convolutional neural network to look for the tombs of nomadic Iron Age rulers by analyzing high-resolution satellite photos of the steppes of central Asia. Other recent projects have utilized machine learning algorithms to identify archaeological sites based on the presence of potsherds or even accumulated heaps of vitrified feces along riverbanks.
Sorting potsherds just got a whole lot easier
Machine learning algorithms excel at spotting traces of the past in digital images and are perfectly suited to do the “grunt work” of sorting and classifying seemingly mundane artifacts, such as broken bits of pottery called potsherds.
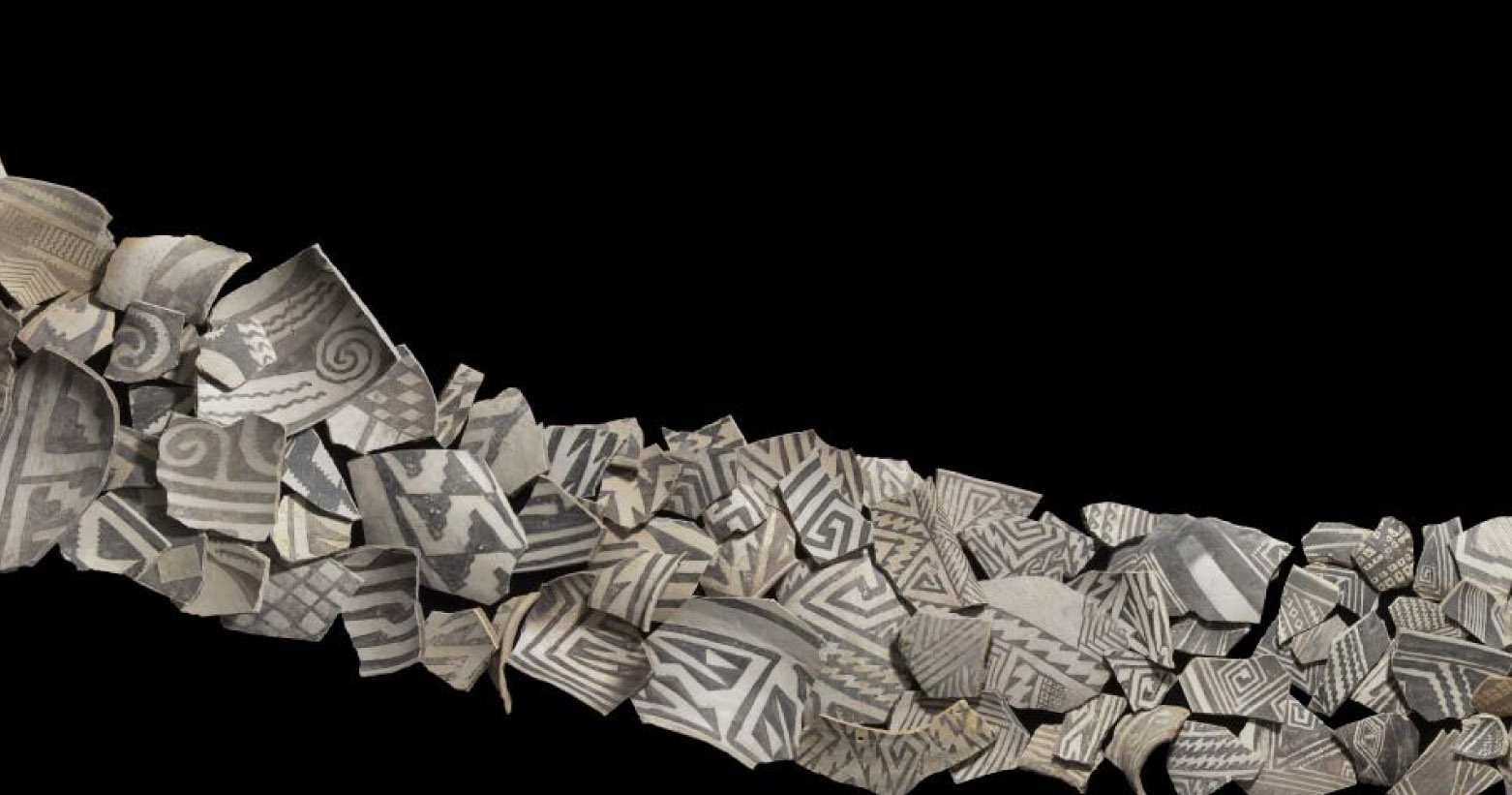
Downum and Pawlowicz trained their algorithm to recognize a style of pottery called Tusayan White Ware, which was made in the American Southwest between 825 and 1300 CE by the ancestors of today’s Hopi people. Photo source: Downum and Pawlowicz, 2019
Northern Arizona University archaeologists Chris Downum and Leszek Pawlowicz recently trained a program to sort potsherds—the bane of graduate students in archaeology programs around the world—because they are both tedious to work with and a crucial part of piecing together the past.
“Recognition of ceramics is still a manual, time-consuming activity, reliant on analog catalogs created by specialists, held in archives and libraries,” wrote University of Pisa archaeologist Francesca Anichini and colleagues in a paper published in November 2020. Anichini’s team developed another application called ArchAIDE, which uses machine learning to classify pottery fragments based on the curvature of the potsherd and how it is decorated.
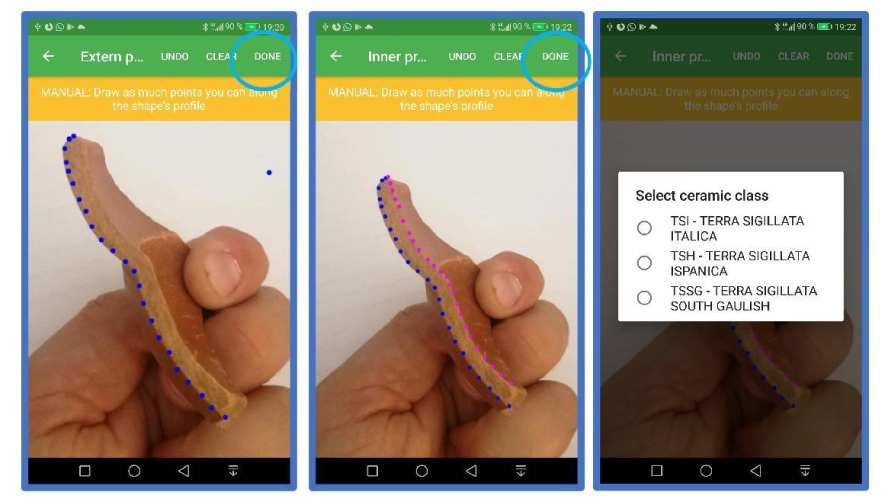
What does identifying a few hundred or a few thousand broken pieces of pottery actually accomplish for archaeologists? In a paper published in August 2021, University of Notre Dame archaeologist Kelsey Reese describes an algorithm that estimates when people lived at an archaeological site based on the types of potsherds left scattered on the ground.
Archaeologists who work in the southwestern US have an established track record of estimating the age of a site by looking at the pottery scattered on the surface—but those estimates are on the scale of centuries. Reese’s neural network, however, can narrow it down to decades.
“The neural network can be much more precise as it considers the actual proportions of ceramic types, and has learned exactly how to weight each of those types in relation to one another,” Reese said. “It provides us with a way to look at population changes and movement with both spatial and temporal precision that has never before been possible.”
AI’s future in studying the past
Broadening the application of machine learning to new areas, time periods, and artifact types appears to be the next move for most of the archaeologists working with the technology.
Altaweel wants to apply his facial recognition algorithm to more common artifact types, like potsherds. With at least three teams working on AI potsherd recognition now, that’s likely to be the next big step forward in archaeology’s adoption of machine learning.
Making the technology more accessible is another common goal. Downum and Pawlowicz envision a mobile application which can be used in the field or in the lab to identify potsherds quickly and efficiently. Altaweel hopes for a similar democratization of the algorithm used to study the faces of ancient statues, as well.
“When someone is in the field doing work, they should be able to instantly compare objects found with other known objects,” he said.
Teaching algorithms to be archaeologists
Whether AI becomes a standard part of archaeologists’ lab and field kits will depend on how accurate and reliable it is. Ultimately, that will depend on the archaeologists who train the algorithms.
In other fields, ranging from law enforcement to college admissions, proponents have touted algorithms as a way to remove human bias from the equation. However, studies have frequently shown that human bias is baked into the very data the algorithms have to learn from.
That’s likely to be true in archaeology as well; in the case of potsherd sorting, for example, archaeologists don’t always agree on what type of pot a fragment came from. That means that if two individuals were building the training datasets, their resulting algorithms might sort potsherds very differently, which would ultimately lead to different conclusions about the past.
To help get around that problem, Downum and Pawlowicz’ training datasets only included examples where all four archaeologists reached a consensus. And they suggested that the algorithm may help archaeologists tackle some of those biases just by being able to explain its decisions. While a human might look at a potsherd and say, “This just looks more like Culture A than Culture B to me,” the algorithm highlighted the specific features in each image that influenced its decision.
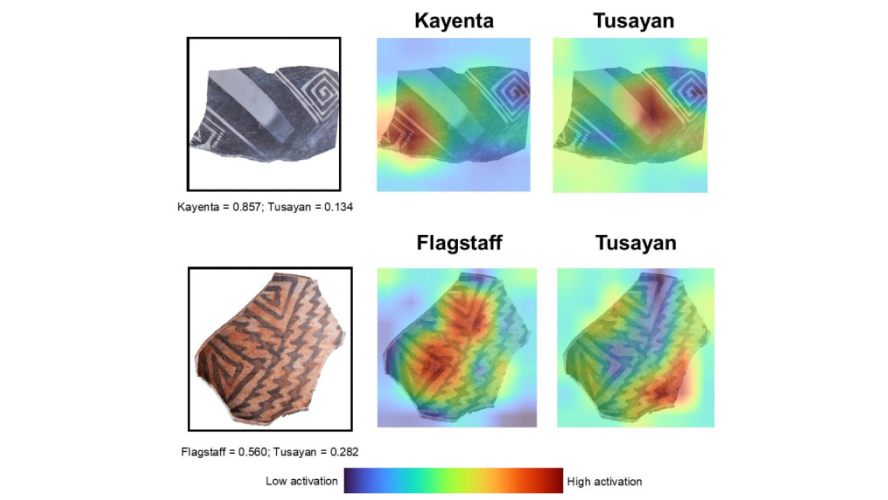
Reese said that depending on the method she used to measure the success of her algorithm, it accurately dated Mesa Verde sites between 90% and 92.8% of the time, a success rate she calls “very robust.” And in a study published late last year, an OBIA algorithm trained to recognize burial mounds in drone photographs of a remote area of Kyrgyzstan was able to detect between 87% and 100% of burial mounds in its search area. Downum and Pawlowicz pitted their neural network against four archaeologists in a sorting showdown. The neural network outperformed two of the humans and tied with the other two.
“I think the advantage is we could use this technology to scale this type of work,” Altaweel said. “So, if I wanted to compare thousands or even millions of objects (assuming you can get that number), it would not be realistic to do it manually. Even for experts this would be a difficult task.”