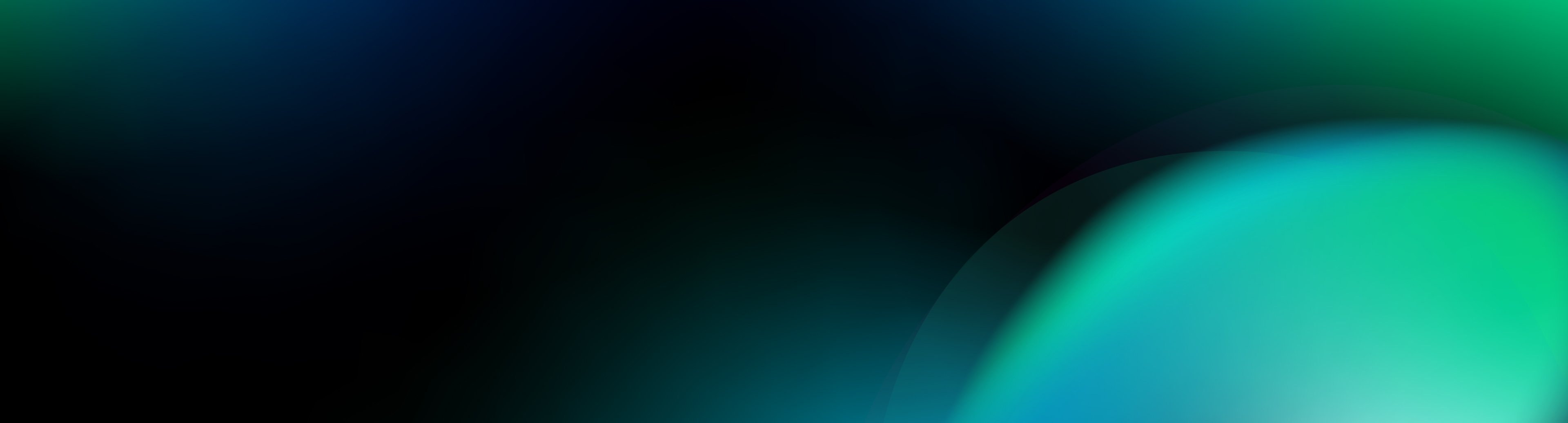
How machine learning is making green energy a reality
The science of machine learning coupled with the traditional laws of physics is helping engineers design innovative approaches to accelerate the adoption of green energy.
The science of machine learning coupled with the traditional laws of physics is helping engineers design innovative approaches to accelerate the adoption of green energy.
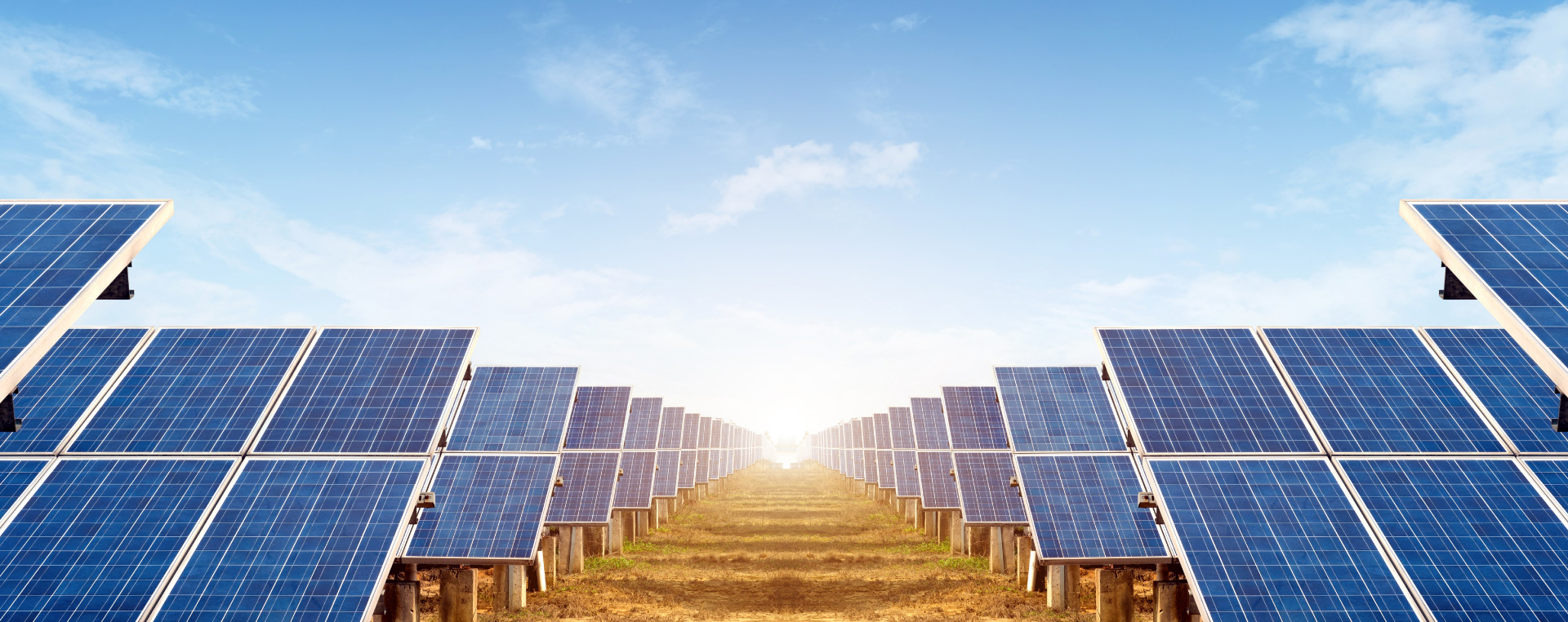
Earlier this year, the MIT Joint Program on the Science and Policy of Global Change published a warning, using data from the Intergovernmental Panel on Climate Change: to avoid a scenario where extreme weather events become the norm, the planet must eliminate greenhouse gas emissions entirely by 2070—better if it can be done by 2050. Reducing emissions to fulfill Paris Agreement pledges will no longer suffice.
EmbraciEmbracing renewable energy is critical to eliminating greenhouse gas emissions. However, the move toward it is anything but easy. The physical constraints of a typical energy network grid hamper the integration of more renewable energy sources. In addition, energy needs for productive and sustained growth in developing regions must be considered to ensure the participation of all countries in meaningful climate action. And investing in green energy is at times still a risky venture, in large part due to market price volatility.
Scientists and engineers are working to solve these challenges and make the universal transition to green energy a reality. One of the solutions that may help make a green future possible lies at the intersection of human creativity and the computational power of machine learning.
Optimizing energy systems with machine learning
On August 14, 2003, a blackout left fifty million people across southeastern Canada and eight northeastern states of the US in the dark for two days. After brushing some overgrown trees, a few high-voltage lines faulted, transferring their power to other lines that in turn shut down from the excess power burden. Like dominoes, lines continued to fail.
Energy grids are fragile, and underlying physical constraints contributed to this catastrophe. Because lines can only carry so much power at any time, a few lines going down can have cascading effects. A generator can’t immediately shift the amount of power it produces; that is a process that takes time to ramp up or down, given the constraints of the generator’s machinery. Furthermore, for the grid to be operational, the amount of power going into the system must equal the amount of power going out at all times.
These constraints pose challenges to the integration of renewables. Their output changes moment to moment with the weather—solar panels generate less energy on cloudy days, and wind turbines can’t spin without wind. Compounding the problem is the fluctuating electricity demand. Given that power into the grid must equal power out at all times against this dynamic backdrop, how do you satisfy the grid’s constraints?
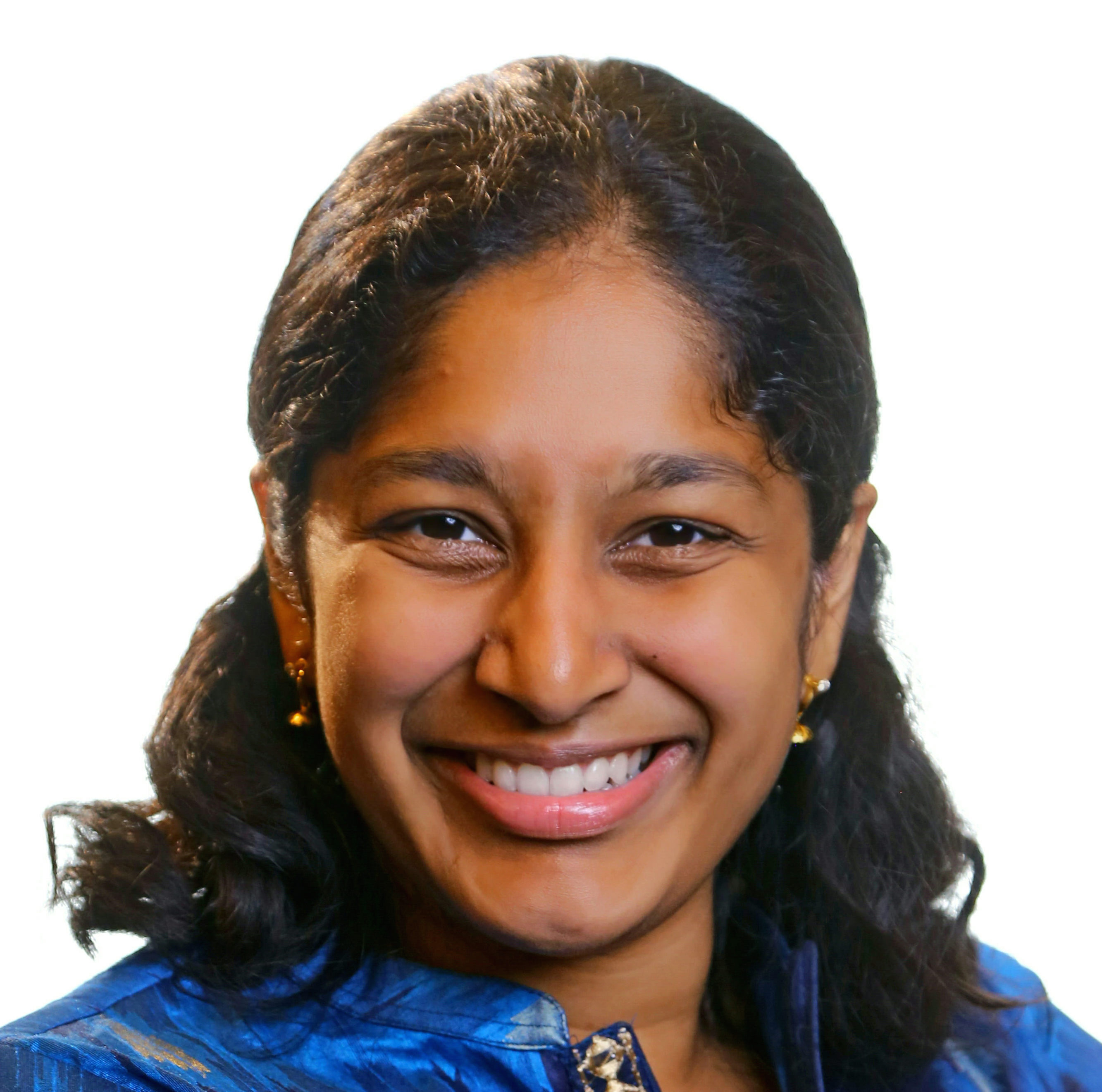
Priya Donti, a PhD candidate at Carnegie Mellon and the chair of Climate Change AI, has proposed several approaches to address this problem with machine learning. “There are a couple of challenges here,” she says. “How do we create better supply-demand forecasts to reduce uncertainty on both sides of the equation? And how can we optimize our electric power systems, given those forecasts, in a way that respects the grid’s constraints?”
As more renewable energy sources join the grid, electric power systems must optimize faster and at a greater scale—faster because output from renewables fluctuates from one moment to the next and at a greater scale because renewables are typically small, meaning there are more devices to optimize. There are two approaches to optimize electric power systems: a centralized approach tackles the problem at the grid level, and a decentralized approach tackles it at the device level.
Existing power-system optimization models are slow because they have to deal with the grid’s physical constraints. Machine learning can speed these models up: “One way is to slim these models down by omitting parts of them. We can use data on historical patterns to find the parts that we can omit,” says Donti.
Through her research, Donti is also exploring whether machine learning can replace existing optimization models altogether while respecting the grid’s constraints. “We’re trying a hybrid approach: combining insights from machine learning with constraints from traditional engineering to ensure that we are aligned with the laws of physics,” says Donti.
In addition, Donti’s work addresses market-based approaches that focus on getting individual renewables, batteries, and consumers on the grid to better control their devices. “Machine learning algorithms can determine when to charge or discharge batteries or how to shift when electricity is consumed, based on what the grid needs at any given moment in time,” says Donti.
Using convolutional neural networks to accelerate electrification in developing regions
Addressing climate change is a global effort. However, the lack of universal energy access to support climate adaptation is a barrier for governments looking to mount a concerted effort to battle the impacts of climate change.
Barriers to grid expansion for energy providers in many countries in Africa include prohibitive connection costs and the data lack that contributes to planning uncertainty. Energy providers need to know which communities don’t have energy access, approximately how much energy consumers would need to build better lives once they receive access, and the best way to connect consumers with constrained resources.
Alternatives to grid extension include solar home systems. These standalone photovoltaic systems can support smaller loads without the need for energy providers to make significant investments in power lines. And in some cases, clustered homes equipped with these systems (and a low-voltage network) can make minigrids viable. Identifying consumption levels is important for energy providers to determine how to boost consumption for economic growth and how to design the best systems for cost recovery.
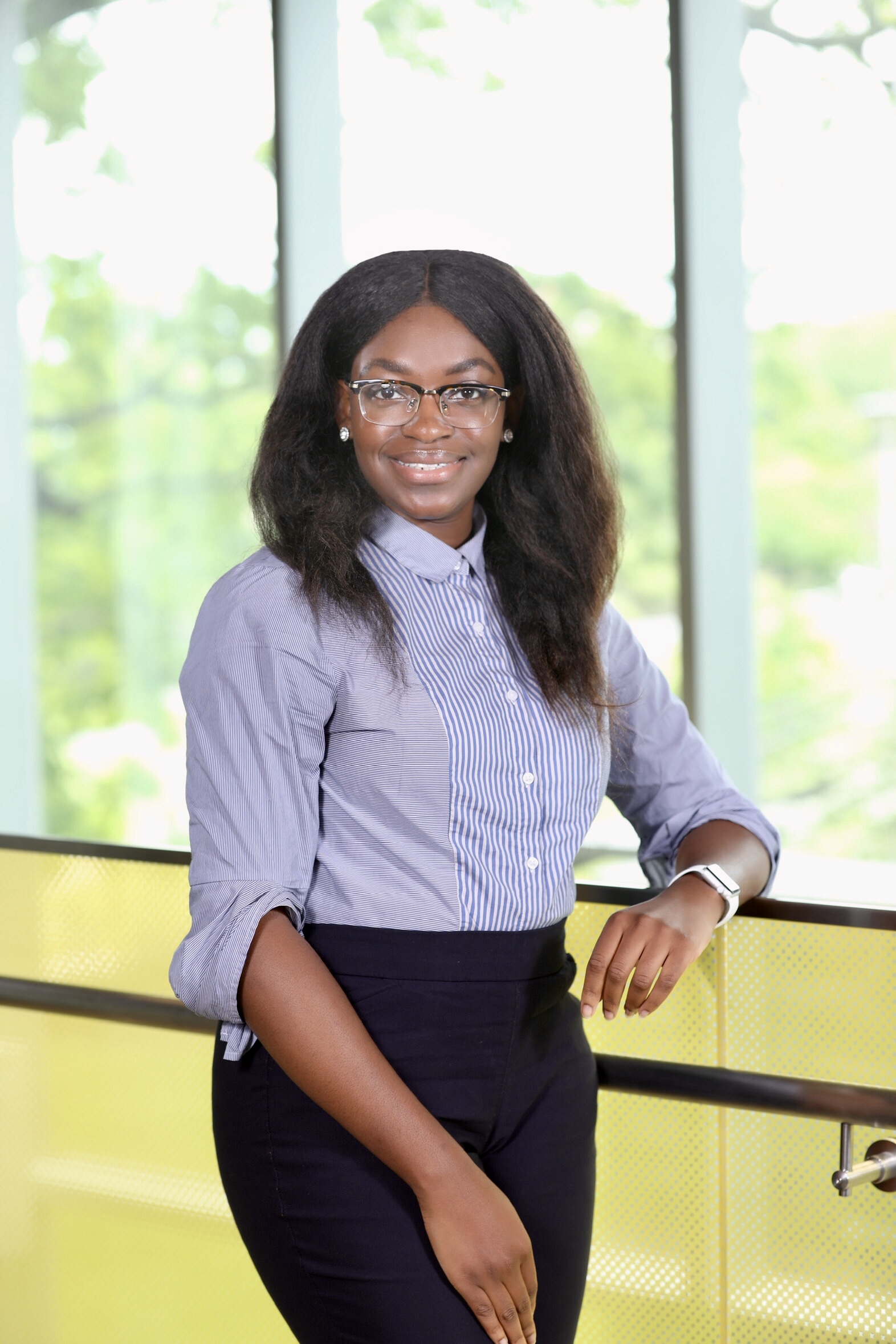
Simone Fobi is a Columbia University PhD candidate affiliated with the Electricity Growth and Use in Developing Economies (e-GUIDE) initiative, a multiuniversity consortium focused on improving electricity system planning and operations in developing regions. She wants to address some of the consumption prediction challenges using machine learning.
“At a high level, we’re using machine learning models to predict future electricity consumption in yet-to-be electrified communities,” she says. “Specifically, we’re applying convolutional neural networks to high-resolution satellite images to make accurate predictions of electricity consumption at the household level in low-access settings.” A convolutional neural network is a type of powerful deep learning algorithm that processes image data to differentiate the various objects and aspects within an image.
Fobi’s work was featured in a 2020 case study by Village Data Analytics (VIDA) and e-GUIDE, “Electricity Demand Estimation and Viability Analysis for Off-Grid Villages in Kenya.” Selecting optimal electrification strategies depends on consumption estimates. For each settlement, e-GUIDE’s Electricity Consumption Prediction Service was used to predict the number of consumers at different electricity consumption levels. The project’s team combined the use of electricity consumption predictions and VIDA’s planning tool to identify and rank remote villages for minigrid suitability.
The Electricity Consumption Prediction Service used daytime satellite imagery and convolutional neural networks to estimate energy consumption of individual buildings in the assessed villages once connected to the grid. “The site suitability analyses work well with e-GUIDE’s building-level consumption predictions to offer energy providers a thorough, precise assessment of how much demand they should anticipate, providing decision support on how best to connect customers efficiently and cost effectively,” Fobi says. Spurring investment in renewables with machine learning–driven price forecasting
Machine learning has commercial applications in the move toward green energy. Consider the work of Boston-based Gaiascope, a software start-up using machine learning to make investment in renewables a more viable option for investors. “One of the barriers to rapid deployment of green energy is a lack of understanding from investors around how to profit from renewables,” says climate scientist and Gaiascope CEO and cofounder Lauren Kuntz, PhD.
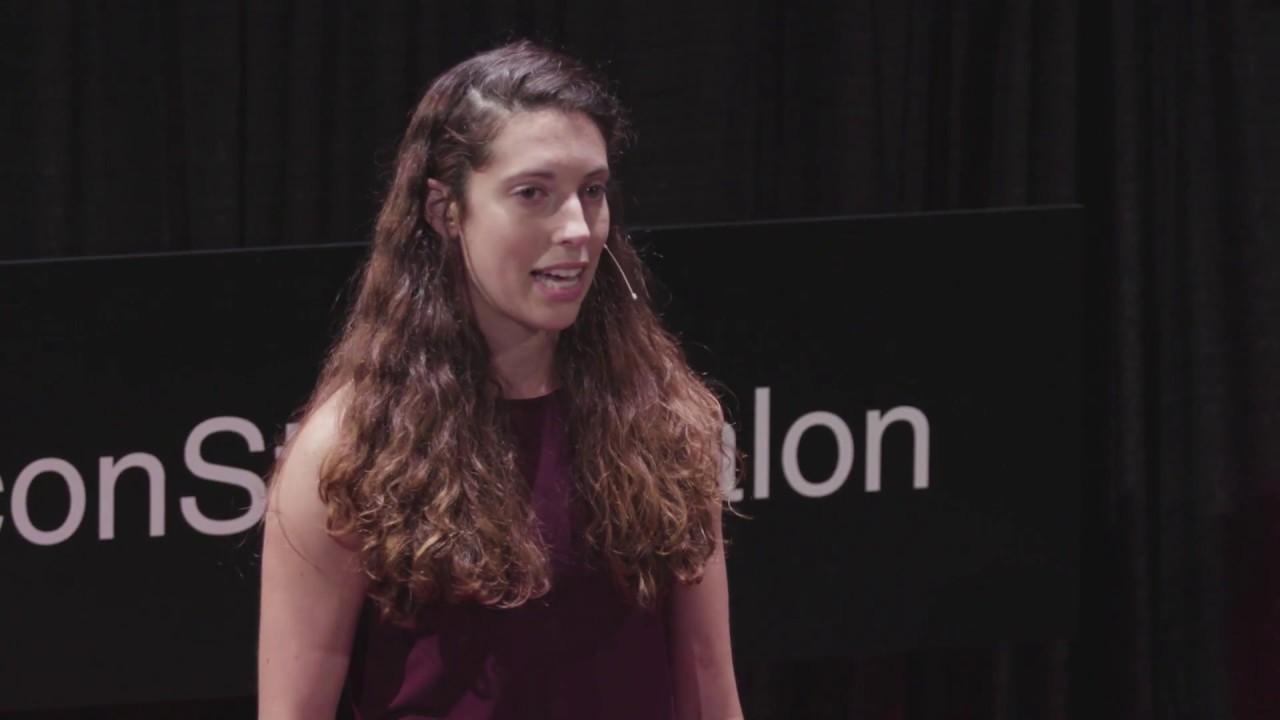
Investors often grapple with the workings of a grid, which at its essence is a dynamic system. Lines go in and out for maintenance, new generators come online, and newly developed residences contribute to increasing loads. Because the price of electricity is tied to supply and demand, which constantly fluctuates, it’s nearly impossible to make accurate pricing predictions. And renewables joining the grid only makes price volatility more severe. Investors that buy into the market without knowing how much energy will cost from one day to the next put themselves at financial risk.
That’s where Gaiascope comes in. Founded in 2019, the company uses machine learning and physical grid models in a hybrid approach to generate accurate price forecasts. The goal is to help energy providers, utilities, and other investors understand how they should position themselves in the energy market. “If you increase understanding of market dynamics, it’s easier for asset owners and operators to maximize revenue from renewables in the short term,” says Kuntz. “And in the long term, increased transparency around price expectations helps investors better evaluate and understand revenue potential and risks. Increasing transparency reduces uncertainty, which helps make green energy a more profitable option.”
Machine learning is a powerful tool helping to move energy production toward a greener, more sustainable future. As machine learning is increasingly used to solve new and existing challenges, it’s important to approach the task with respect for the variable nature of physical energy systems. “There’s always a temptation to ask, ‘Where can I find a dataset, and what algorithm can I run on it?’ That’s not how you approach it,” says Donti.
Power grids have the same physical equations all around the world, but no two grids are the same. Each has its own topology and market structure governing it. Some will be centralized while others decentralized. According to Donti, “My advice to future stakeholders is to get boots on the ground; understand the needs, barriers, and complexities of the energy systems they work on; and develop, apply, and refine their machine learning models from the bottom up.