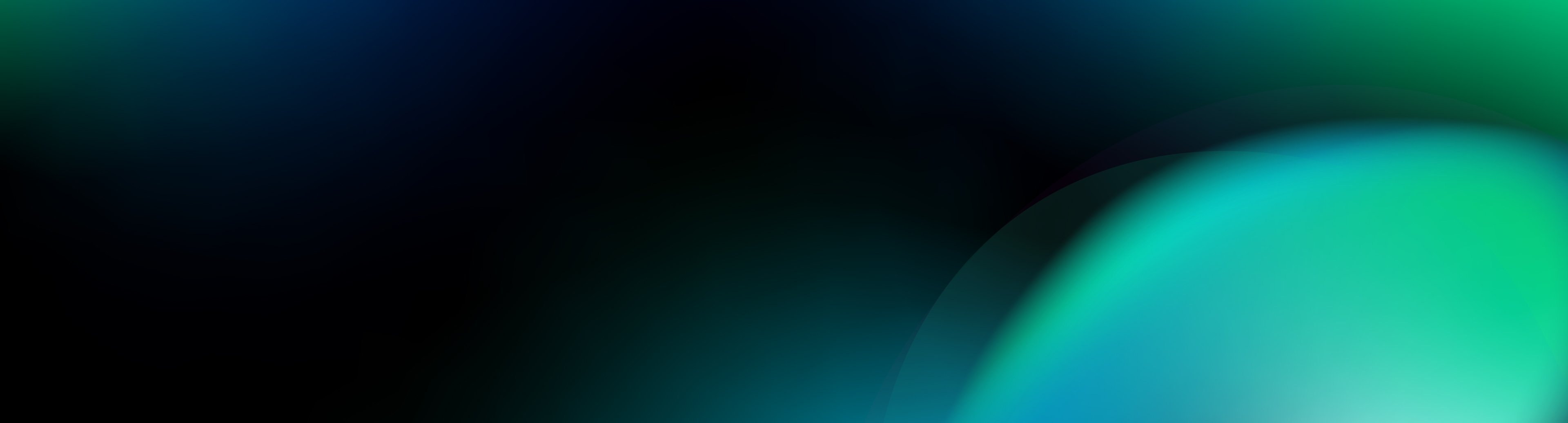
A transformative breast cancer treatment that works for patients across multiple ethnicities and countries
Meet Adjutorium, a powerful new algorithm that can help physicians identify the patients that will benefit most from adjuvant therapy.
By Kayt Sukel
By Kayt Sukel
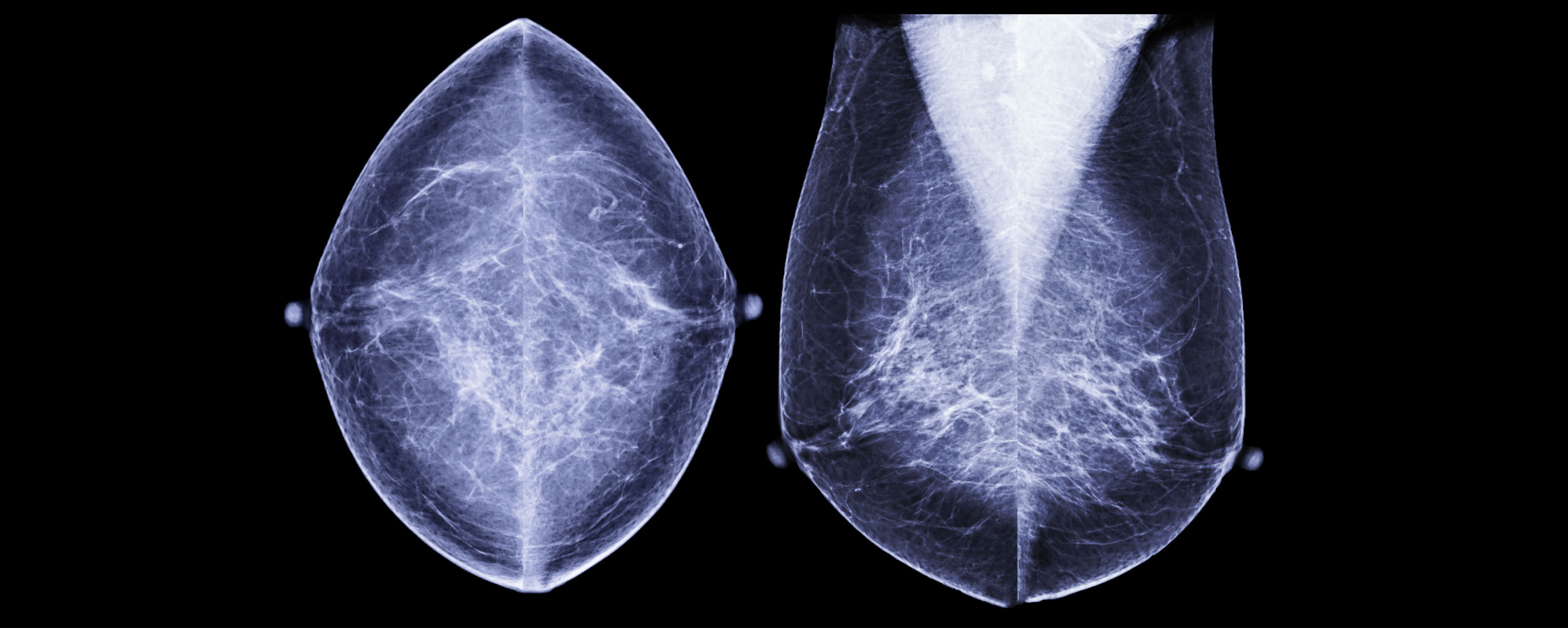
A machine learning algorithm is being developed to chart a course for adjuvant breast cancer therapy. Photo source: Shutterstock
The doctor looks over the characteristics of their patient’s breast tumor. It’s a larger invasive carcinoma, and the tumor has tested negative for estrogen receptors, progesterone receptors, and excess human epidermal growth factor receptor 2 (HER2) protein. The doctor will recommend breast-sparing surgery to remove the triple-negative tumor, but they now have to make a call about whether or not to recommend adjuvant therapy, an adjunct treatment like chemotherapy, hormone therapy, radiation therapy, or immunotherapy, to help bolster the surgery’s effectiveness in battling the disease.
Dr. Valerie Vanderweerd, an obstetrician at Belgium’s University Hospital Leuven, says that it is a decision that will have profound implications for the patient’s ultimate outcomes.
“Sometimes the pathology offers us a straightforward answer regarding adjuvant therapy. For example, we often see low-grade estrogen receptor positive tumors that benefit from hormonal therapy. But given that we have to balance the side effects of adjuvant therapy and we don’t want to overtreat patients, there are other cases where it is not always clear that adjuvant therapy is the answer. It is not always easy to determine whether it will help.”
In 2020, there were more than 2 million people diagnosed with breast cancer. With screening programs and a host of improved therapies, the disease has a 90% relative survival rate. However, an individual’s treatment outcomes depend greatly on doctors choosing the most appropriate therapies at the right times, a series of decisions that include adjuvant therapy.
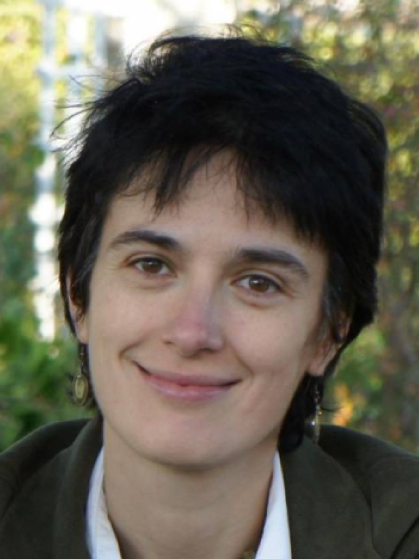
Mihaela van der Schaar, the John Humphrey Plummer Professor of Machine Learning, AI, and Medicine at the University of Cambridge, believes that machine learning algorithms can help improve the outcomes for breast cancer patients.
“This is a decision that can lead to extra years of life, as well as a higher quality of life for women diagnosed with breast cancer,” she explained. “While there are statistical models that can help doctors make this decision, due to a lack of adequate training data, they often fail to predict the right treatment for certain subclasses of patients, like younger women, and women of African descent. In these cases, we can use technology to help doctors make better, more informed decisions about which treatments to pursue.”
Treatments for all ethnicities and subgroups
Today, many clinicians have access to statistical models to assist with clinical decision-making. These include Predict Breast Cancer, an online tool built by the Cambridge Breast Unit, the University of Cambridge Department of Oncology, and the United Kingdom’s Eastern Cancer Information and Registration Centre.
Predict analyzes a host of factors, including the size and type of breast cancer, whether the disease has spread to the lymph nodes, and whether the tumor expresses markers indicating it is estrogen, progesterone, or HER2 positive, among others, to recommend treatment options.
Dr. Jem Rashbass, former Executive Director of Data and Analytical Services at Great Britain’s National Health Service (NHS) Digital, said this decision-support tool has been very helpful. However, its efficacy has been limited by older data, as most clinical trials are conducted years before publication. In addition, there is often a paucity of data for smaller subgroups of patients.
“There are limitations on utilizing this kind of statistical approach to the analysis of population data,” he explained. “You can’t get to a very fine level of granularity on small subgroups of patients, who we know don’t benefit from the standard course of treatment. These kinds of models are fine for dealing with white women of a certain age that have been diagnosed with breast cancer. But when you get into ethnic groups or different age groups, the number of cases you’ve got in an original dataset simply is not rich enough to extract meaningful learnings.”
To provide actionable insights into patients in smaller subgroups, van der Schaar and colleagues have developed a new machine learning–driven prognostic tool called Adjutorium. Van der Schaar said she was inspired to develop the tool, one of several machine learning tools to help with breast cancer treatment decisions, because of her own experience with breast cancer: her mother succumbed to the disease when she was younger.
Unlike traditional statistical models like Predict Breast Cancer, Adjutorium uses an entirely different approach, analyzing data about individual patients in a nonlinear fashion to assess whether adjuvant therapy will offer improved survival rates and other treatment benefits.
“The tool is based on a state-of-the-art automated machine learning framework that learns from the data. It lets us know about nonlinear interactions that may exist between different data features,” she said. “These interactions are complex—and what’s nice about this approach is that it learns on the basis of the data we give it. It not only allows us to see differences across different subgroups of women but, in the future, we can also add in new information, like genetic data, social determinants of health, nutrition data, or other outcome information to make it even more up-to-date and powerful.”
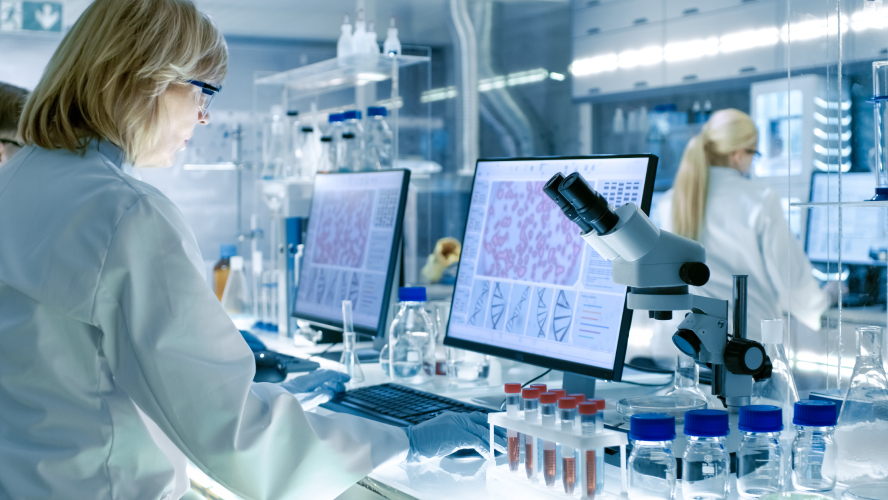
Van der Schaar said that the field of medicinal research is constantly evolving as different nuances of different types of breast cancer and associated treatments come to light every day. Machine learning algorithms need to be able to integrate that information to evolve. Rashbass agrees.
“What machine learning does is allow us to take these prediction models to the next level,” he said. “We can interpret much smaller datasets much more rapidly. The challenge with so many datasets is that, particularly with a disease like breast cancer where long-term survival is actually quite good, you need long-term datasets to help you see what is going to happen to someone ten years after they’ve been treated.
“The people you are treating today are not being treated in the same way as patients were treated ten years ago. Statistical models don’t have the ability to help you understand the outcomes for people who are being treated with new therapies. Machine learning, which can extract data from much more complex and rich datasets, can help you provide better recommendations.”
This approach has proven to be generalizable, too. Adjutorium, trained on an NHS dataset from the United Kingdom, was recently validated in the United States.
“Most machine learning methods only look at one country or one population,” said van der Schaar. “Here we look at two different populations and see if we can generalize to another country. We do that by building machine learning methods that are well-calibrated to the new data and population.”
Driving improvements to machine learning
Van der Schaar says that machine learning algorithms will not help further healthcare—however, developing solutions for healthcare can further the state of the art in machine learning. And it will do so across a host of different medical conditions.
“We’ve developed a similar tool for cardiovascular disease, and we discovered that diabetic patients and non-diabetic patients have different needs,” she says. “We also found that there were also important differences between males and females. What’s nice about this technology is that it can learn what features and variables are most important for different subgroups within a certain disease, how those features interact, and what that means for treatment options.”
To that end, van der Schaar hopes that business leaders will facilitate partnerships between scientists like herself and clinicians so they can build useful, usable tools to improve patient outcomes across a variety of different diseases.
“Technology companies all over the globe are pushing technology on clinicians and patients that may not truly be useful for them,” she said. “When we can have true partnerships, where we work together, we can drive technology that is beneficial. It takes time and energy to understand one another, and what machine learning can accomplish. But it’s what we need to move forward.”